Multi-Sensory Feedback for Myoelectric Control
Our objective is to investigate novel multi-sensory feedback approaches to improve muscle control for greater independent function or better control of a myoelectric assistive device
Isometric Muscle Control of a Virtual Myoelectric Prosthetic
Using a machine-learning algorithm, isometric muscle activity is mapped to directional intent for controlling a virtual myoelectric prosthetic. A transparent arm helps guide the participant by displaying the shortest path length between targets. The photo below is of a clinical participant with SCI completing our brace protocol at the VA hospital.
Upper-body Restrictive Brace for Virtual Reality Rehabilitation
The major platform for our multi-sensory feedback research is an upper-body restrictive brace that integrates with a virtual reality environment. The brace is designed to restrict motion at the elbow and shoulder in any desired arm position for isometric muscle training. Isometric muscle training is used following neurotrauma and improves independent function (after the brace is removed) or myoelectric control of a remote device (prosthetic, teleoperation). The brace is designed in a Computer Aided Design program (LEFT) and can be ported over into a virtual reality environment (RIGHT) to demo how the electromyography sensors (RIGHT, red) and vibration motors (RIGHT, blue) would properly integrate with the brace.
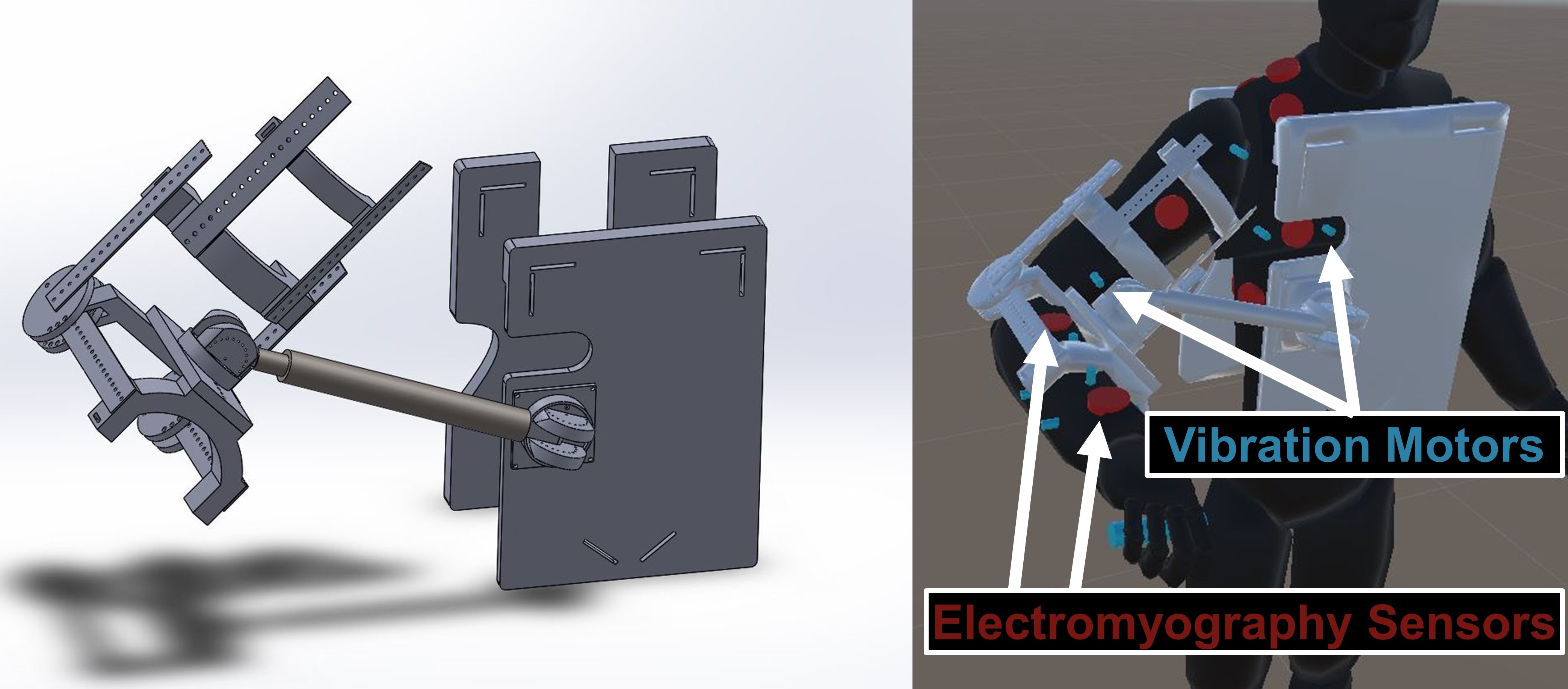
Visual and Haptic Feedback for Virtual Reality Based Isometric Training
While donning the restrictive upper-body brace, subjects control a virtual prosthetic to complete a point-to-point reaching task. Visual feedback is provided as a 'ghost-arm' that is an identical representation of the subjects prosthetic but presented in a transparent material that follows the shortest path between the starting position and desired target. Vibrotactile feedback can guide subject position relative to the shortest path by mapping vibration magnitude to position error magnitude. Vibration can also alter neurophysiological signals and user proprioception for more cognitive-based approaches to improve muscle control.
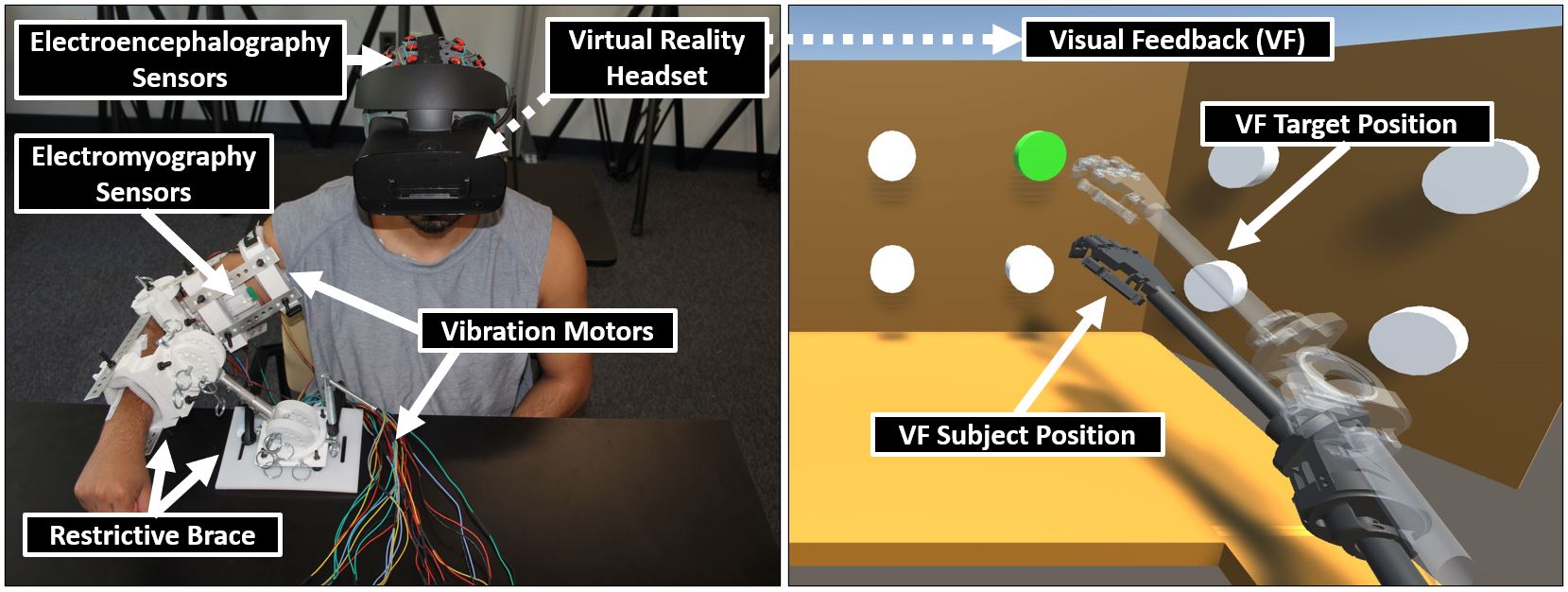
Machine-Learning Classification of Reduced Muscle Sets
A virtual reality task was developed that required subjects to induce isometric muscle activity for controlling an object through a 2D maze. Different machine-learning classifiers, ranging from linear discriminant analysis to neural networks, were developed for mapping electromyography activity to directional intent. Additionally, multiple reduced muscle sets were evaluated by reducing the number of electromyography inputs into the classifiers to 'replicate' neurological disorders or neurotrauma. The combination of various muscle sets and various reduced muscle sets were evaluated for the effects on task performance (completion time, number of wall collisions) and classification accuracy. K Walsh et al., February 2021, doi: 10.1016/j.bspc.2021.102487.
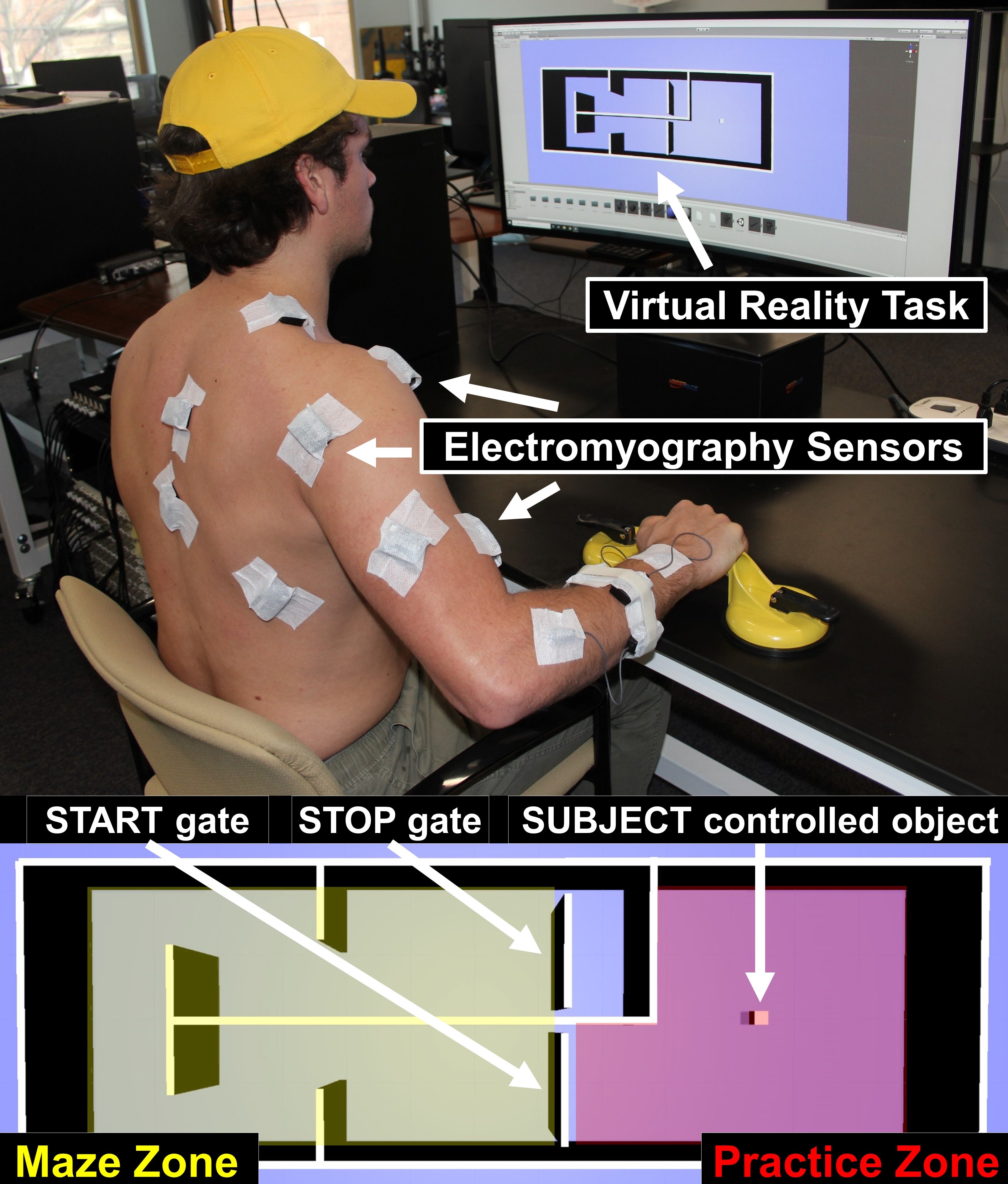
Visual Feedback for Rehabilitation and Movement Assistance Training
Visual feedback (VF) can be presented in a myriad of modes, depending on the timing, style of display, and performance metric objectively targeted. VF outperforms audio and haptic feedback for training user spatial positioning of complex motor-tasks [Sigrist 2013]. Our interests are in developing VF paradigms that effectively train the user to complete a dynamic rehabilitation task for more effective assist device control.
Visual Feedback Training for a Two-legged Squat Exercise
VF was presented in a variety of forms for training the subjects on a dynamic rehabilitation task. The objective was to determine the visual feedback mode with the greatest reduction in movement error over a single training session. The winning VF trained the greatest consistency of spatial positioning and muscle activation patterns. S Sanford et al., February 2021, doi: 10.1123/jsr.2020-0234. S Sanford et al., May 2020, doi: 10.1080/00222895.2020.1770670.
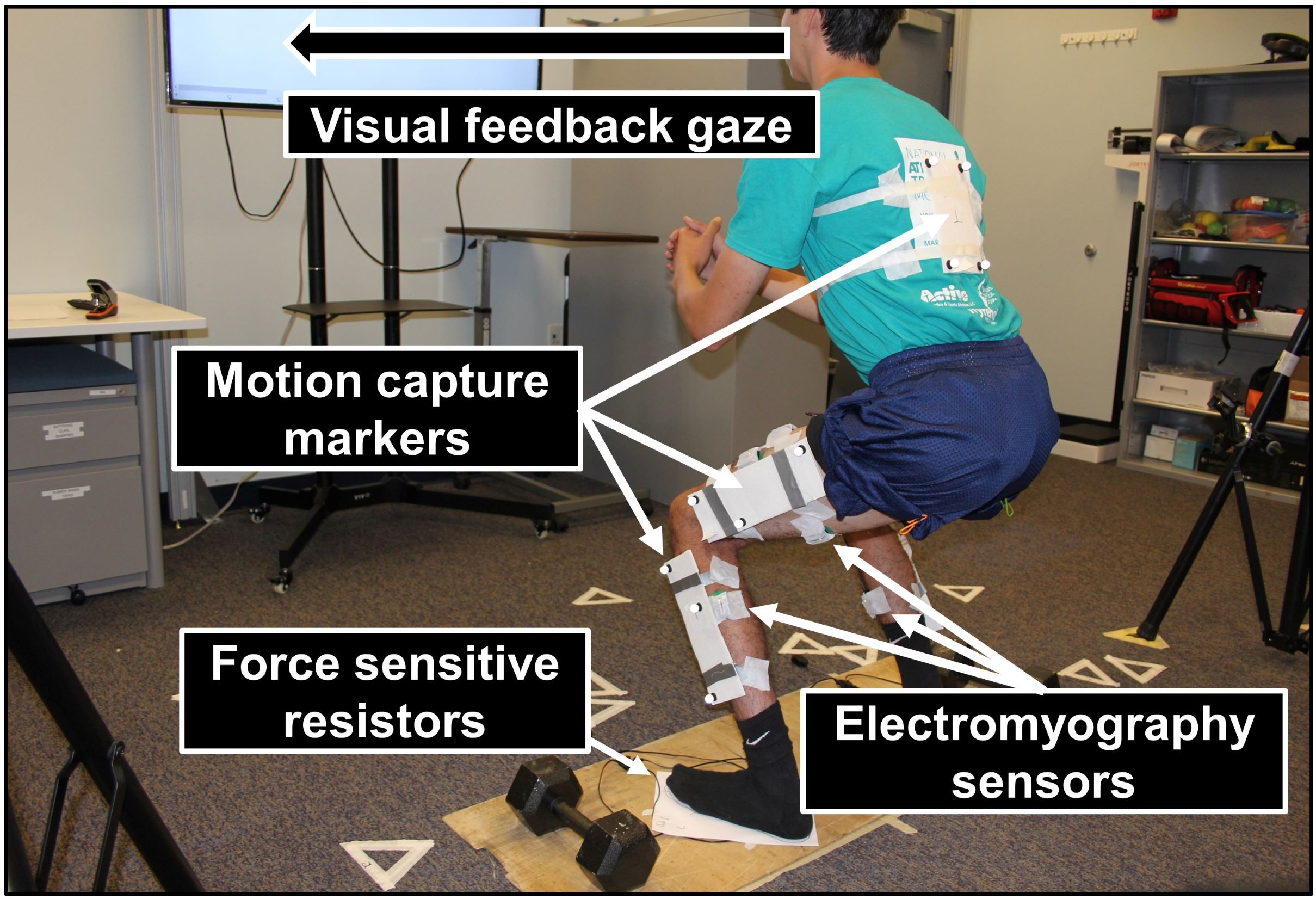
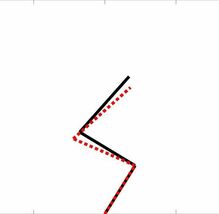
Rectus Femoris Muscle Activation Influenced by Changes in Squat Technique
The two legged squat exercise is clinically correlated to the sit-to-stand movement. Muscle activation patterns are highly influenced by technique and are captured in real-time by surface electromyography sensors on lower-limb muscles primarily responsible for force generation. Training the user to do a complex rehabilitation task in a more consistent movement pattern will induce consistent muscle activation patterns. The video below is a demonstration of electromyography activity of the left and right rectus femoris muscles during different depths of the two-legged squat exercise. Our objective is to evaluate different visual feedback features for optimizing movement rehabilitation training.
References
1. S Sanford, M Liu, T Selvaggi, R Nataraj, “Effects of Visual Feedback Complexity on the Performance of a Movement Task for Rehabilitation,” Journal of Motor Behavior, May 2020, doi: 10.1080/00222895.2020.1770670. 2. S Sanford, M Liu, R Nataraj, “Concurrent Continuous Versus Bandwidth Visual Feedback With Varying Body Representation for the 2-Legged Squat Exercise,” Journal of Sport Rehabilitation, February 2021, doi: 10.1123/jsr.2020-0234. 3. R Sigrist, G Rauter, R Riener, P Wolf, “Augmented visual, auditory, haptic, and multimodal feedback in motor learning: a review,” Psychon. Bull. Rev., vol. 20, no. 1, pp. 21–53, Feb. 2013. 4. K Walsh, S Sanford, B Collins, N Harel, R Nataraj, “Performance potential of classical machine learning and deep learning classifiers for isometric upper-body myoelectric control of direction in virtual reality with reduced muscle inputs,” Biomedical Signal Processing and Control, February 2021, doi: 10.1016/j.bspc.2021.102487.